Hello DALL-E!
TL;DR: I let my friend Ava (who actually knows a thing or two about art!) experiment with DALL-E 2 for a bit. She allowed me to share her reflections with you! Enjoy!
Meta: guest post by Ava Kiai, a very awesome individual who works with bats in her PhD and who knows a thing or two about art! Lots of pretty pictures in this one!
DALL-E is a powerful little parlor trick in your pocket. But what can it do? In what way does it constitute an advance? What is it good for? And what does it mean for the future of AI’s integration with how we live and work? With the help of a couple of friends, I tried to find out.
The past decade has brought about so many varied and surprising advancements in AI that expressing astonishment at the capabilities of new AI algorithms has begun, in some circles, to feel a bit passé. But show any of these new models to a layperson and it becomes clear that we have not yet outgrown Arthur C. Clarke’s adage that “any sufficiently advanced technology is indistinguishable from magic.”
The two leading competitors in AI development, DeepMind (founded 2010) and OpenAI (founded 2015), have been engaged in a public battle to make AI models increasingly more intuitive, intelligent, and, at a somewhat delayed third place, aligned with human interests. OpenAI seems to have won the most recent skirmish1 with DALL-E and DALL-E 2, a neural network model that can generate images de nuovo from plain-language text prompts, and now also modify and improvise on existing images.
The terminally-online have almost certainly encountered viral images generated by DALL-E that illustrate alternately its remarkable ability to produce rich images from simple prompts and its quirkiness when asked to generate unusual images. But perhaps the most famous image associated with DALL-E thus far, and now reminiscent of the early, innocent days of prompt engineering2, is that of “an illustration of a baby daikon radish in a tutu walking a dog.”
There are many articles online explaining how it works, in addition to OpenAI’s own press releases and blog, so I will not discuss this very much here. Rather, I want to attempt some answers to questions that kept turning in my mind as I began playing with DALL-E and as I spoke to friends both savvy and naive about the current state of AI: Why is this technology impressive and how can this be made more apparent to a layperson? And, perhaps most importantly, what’s it good for?
Here it goes:
Why is this technology impressive, and how did we get here?
At its core, DALL-E is a computer algorithm that can take any request in plain language and produce an image of what was asked for. This is, for many people, no less baffling than if you handed them a magic lamp and told them they need only rub it and out will emerge a genie that can answer any prayer.
More practically speaking, DALL-E, its descendants and its competitors, have put on display the giant leap forward that artificial intelligence has made in the past decade.
In the not-so-distant past (in 2016 to be exact), the cutting edge of AI already included algorithms that could generate images from text3. That cutting edge produced the following images from the prompt, “this magnificent fellow is almost all black with a red crest, and white cheek patch”:
Today, the same prompt given to DALL-E produces considerably improved results.
DALL-E has “learned” the relationship between hundreds of millions of text and image “tokens” from all over the internet. When asked to produce an image, it draws upon this extreme breadth of “experience,” and astonishingly, exhibits what appears to be common knowledge about the things it’s being asked for.
One of the most striking things about DALL-E’s generations is the amount of detail that the model is able to infer from the prompt, but which is not explicitly stated. This includes visual features such as semantically or contextually-related objects (such as the grass and tree branches above), shadows, perspective (such as the birds viewed from below if they are perched on trees but viewed from an equal or higher plane if standing on the ground), distortion, and so on.
Most charmingly, DALL-E can produce images in any “style” or texture asked for, and incorporate rich amounts of detail in keeping with that style.
Thus, when prompted with “a Cubist painting of a bat painting a self-portrait at an easel”, DALL-E returns an image with the angular shapes and shadows typical of Cubist painting. When prompted to return “an Impressionist painting”, it produces images with visible, broad brush-strokes, with shading and light details that are too unmistakably “impressionistic”. (Note that in the examples below, some prompt engineering and cherry-picking were employed.)
“[DALL-E generates] images that, to many of us, feel 'artistic' in some meaningful sense.”
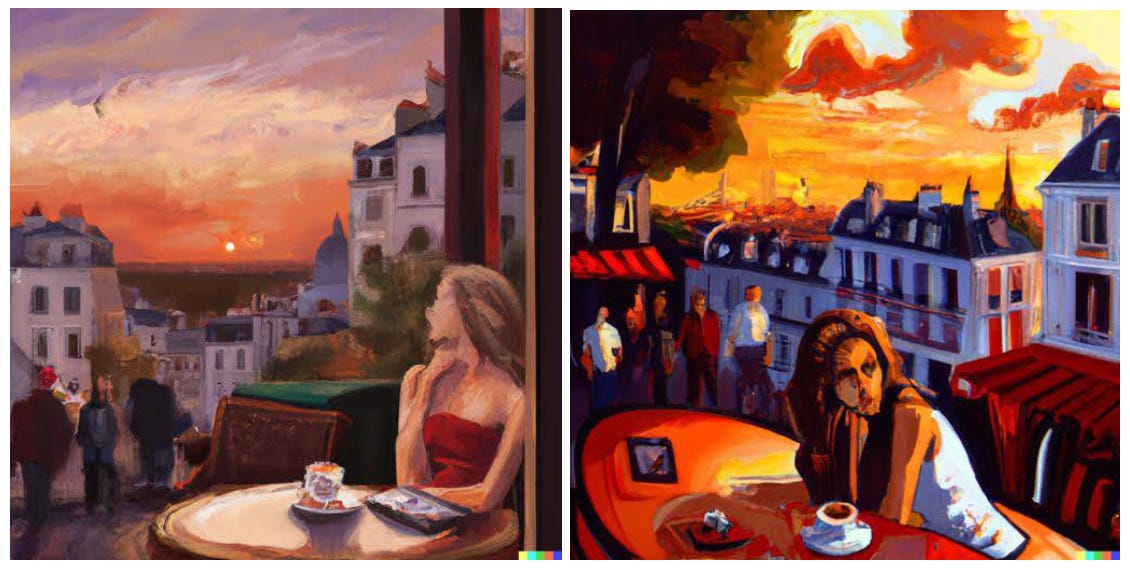
“DALL-E 2 really impressed many of us in terms of the convincingness of the model's generations. [DALL-E generates] images that, to many of us, feel 'artistic' in some meaningful sense.”, says Peter Harrison, Director of the Center for Music and Science at the University of Cambridge, who uses computational tools to study music perception. ”This feels like a particularly important phenomenon in that (unlike with GPT-3's text generation) the output is artefacts that the user could not, in any practical sense, produce through conventional means, at least not without extensive training in artistic techniques (e.g. drawing and painting).”
Take as another example a prompt asking for zebra finches at a farmer’s market in the style of the Japanese artform Ukiyo-e. Not only are various fruits pictured at different planes extending backwards from the viewer, DALL-E also portrays the finches at close perspective, a feature typical of the form. In one adorable image, the finches appear to be taking a break from their shopping to enjoy a small lunch of finch-sized fresh fish.
These and other examples highlight abilities that were largely absent (or were far more primitive) in previous iterations of image generators4.
As others have noted, DALL-E struggles somewhat with combining concepts, called “compositionality”. An example would be asking for one object sitting on top of another.
Of course, it can get many “simple” compositional tasks right, such as generating a “red boat” (where red and boat comprise a unified concept) or even generating fantastical chimeras. Yet, some seemingly simple prompts flummox the engine. It can also entirely neglect parts of the prompt56.
Here, it may be useful to say a few words about DALL-E’s underlying architecture. While there are many moving parts behind DALL-E, its success is due in large part to the breakthrough development of GPT-3, the incredibly successful natural language processing (NLP) algorithm7.
GPT-3 not only smoothly understood and generated text, but also demonstrated that ordinary language - the way that we speak it - can effectively be used to instruct machines to perform tasks. The texts that GPT-3 can generate, after some mild editing, are surprisingly convincing8 and even made it to the op-ed pages of The Guardian9. With some intermediate steps, GPT-3 was used to leverage text as the interface for image generation.
“Text is really the ultimate user interface; you don't need to teach the user any controls or custom programming language, you just tell them to instruct the model what to do in their own words, ” says Harrison.
Like most things in tech, the wild improvement in the quality of these models can also more simply be attributed to increases in the size of the dataset and in computing power. For machines and militaries alike, quantity has a quality of its own.
While DALL-E can sometimes struggle to integrate all components in the prompt, it can also surprise you with what appears to be real-world knowledge of historical context and artistic convention. For example, when prompted with “a Byzantine mosaic”, one of the results features a distorted image that seems (to me) to closely imitate the perspective distortion induced when viewing from below a mosaic constructed upon the inner surface of a dome.
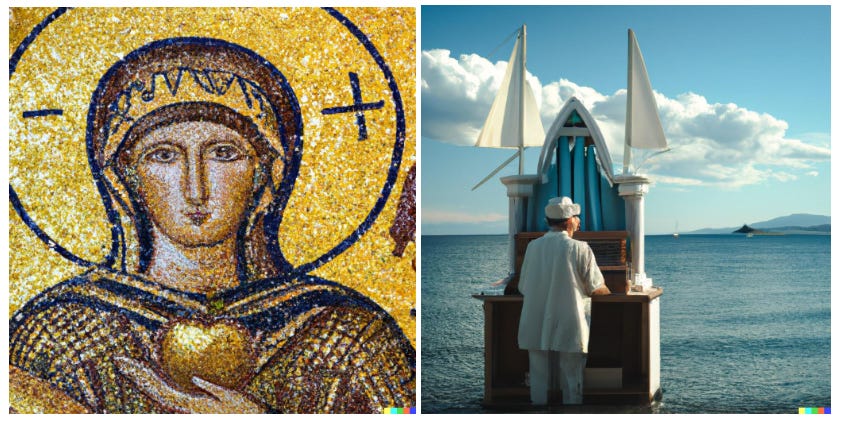
There are (at least) two levels at which we can understand what DALL-E means for AI: the first is at the level of the current implementation: What is the import of a flexible text-to-image or image-to-text generator? The second is at the level of the underlying mechanism: What does this advance in natural language and image processing enable us to do?
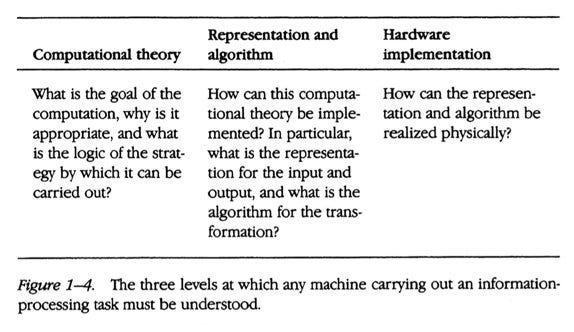
At the level of the text-to-image implementation, DALL-E (and its descendants) offers a[n inverted] solution to a problem that is central to building intelligent and flexible AI. Namely, how do we get machines to understand images? The impact of this is wide-ranging, and already ubiquitous in the form of face recognition technology on your phone.
Of course, currently, DALL-E shows us the opposite: how a machine can understand written language and generate an image. This itself constitutes an important step towards building machines that can relate textual and visual semantics to an arbitrary level of sophistication and flexibility. As OpenAI states, “[DALL-E] helps us understand how advanced AI systems see and understand our world, which is critical to our mission of creating AI that benefits humanity.”
At the level of the underlying mechanism or, to borrow from David Marr’s framework, the computational theory, DALL-E demonstrates an ability that has long eluded AI researchers: how do we get a machine to understand something it has never seen before?
DALL-E’s ability to create beautiful chimeras and fantastical images is certainly entertaining. But what truly constitutes progress in AI is that here, an algorithm can understand and produce a response to a command that was never explicitly part of its training set. What’s more, it can do so even when the command is given in plain, non-specialist language. Intriguingly, this ability mirrors the central insight that spurred the cognitive revolution in experimental psychology, typically attributed to Noam Chomsky, that children can produce sentences they never actually heard.
This is the ideal embodied by the robots in Star Wars, which have no problem understanding and interacting with humans, even when they don’t express themselves in complete sentences.
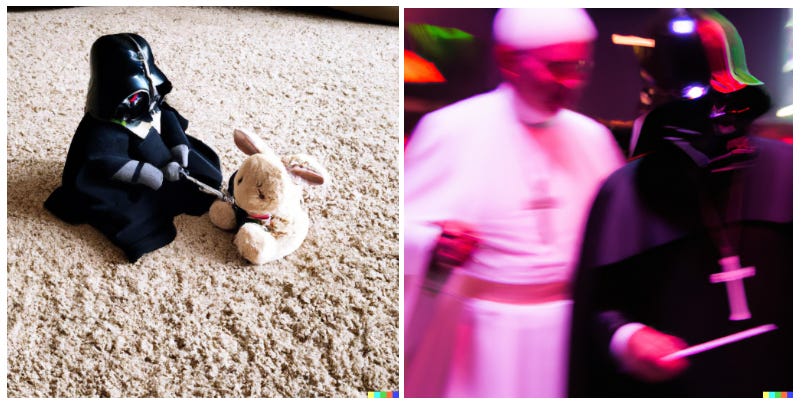
But what’s it good for?
As I was testing out what DALL-E can do well and what it can't, I found myself often wondering what practical application it may have. Can this develop into a tool whose utility extends beyond entertainment? Can it be more than a souped up parlor trick?
Perhaps a good place to start would be to consider OpenAI’s own recommendations. The company is very non-committal regarding possible applications, saying on their Github,
“While we are highly uncertain which commercial and non-commercial use cases might get traction and be safely supportable in the longer-term, plausible use cases of powerful image generation and modification technologies like DALL·E 2 include education (e.g. illustrating and explaining concepts in pedagogical contexts), art/creativity (e.g. as a brainstorming tool or as one part of a larger workflow for artistic ideation), marketing (e.g. generating variations on a theme or "placing" people/items in certain contexts more easily than with existing tools), architecture/real estate/design (e.g. as a brainstorming tool or as one part of a larger workflow for design ideation), and research (e.g. illustrating and explaining scientific concepts).”
The first (and most banal) application that comes to mind is its use for generating graphical content. It’s not difficult to imagine that future versions of DALL-E could become sufficiently good at understanding the task that they may compete with human graphic designers. But while DALL-E may democratize access to bespoke graphic images (e.g. a layperson’s ability to generate a fitting logo for their website), it seems unlikely that even an army of DALL-E’s would unseat the human element in art and design.
AI art, like forgeries, are artifice, not art, for they lack the most compelling aspect of the process of creation: original intent.
“It might be that, with time, we become less impressed by the algorithm's ability to emulate the immediate stylistic impressions of particular artists, and care more about other abilities that remain (for now) the domain of the human artist, for example conceptual creativity or stylistic innovation,” Harrison adds.
AI-generated art can be interesting, novel, or appealing. But AI art, like forgeries, are artifice, not art, for they lack the most compelling aspect of the process of creation: original intent. We protect, maintain, and on occasion spend exorbitant amounts of money on originals, and care little for copies. We feel deceived if we discover that objects we believed to be the work of the master, like Shakespeare’s first folios or Rembrandt’s studies, turn out to be the work of a conman, or even just an apprentice.
These tools are already becoming ubiquitous. The challenge will be to figure out how to negotiate our relationship with them. A plausible middle ground between the full automation of graphic design and the rejection of AI’s influence in artistic domains may lie in the integration of these tools into the designer’s toolkit, just as some designers already use image generators in-house to assist or speed up prototyping.
Turning to more nefarious uses of ad libitum image generation, some readers might wonder, Won’t this make it easy to create “deep fakes” of people doing incriminating things? What about its potential for generating harmful images? We already have a misinformation problem, won’t this make it worse?
Here’s the corporate answer: Such use is strictly prohibited by OpenAI, and the development team is actively working on ways of detecting subtle uses of its algorithm that constitute content violations.
But if we’ve collectively learned any lesson from the period 2016 - 2021, it’s that social media is not to be trusted and its developers even less. Content filters are never solutions, only trade-offs. We will never be able to completely pre-empt the use of these tools for dubious ends. Rather, it will require the continuous re-tuning of our intuitions. It may be that people have already developed a healthy suspicion of what they see online. As these tools become distributed across the internet and in our daily lives, that internal detector will need constant maintenance.
Harrison again: “When early photographers experimented with staged effects (e.g. the Cottingley Fairies) many viewers were convinced as to their veracity, but later generations developed much better abilities to distinguish real from fake images. It might be that, with time, we become less impressed by the algorithm's ability to emulate the immediate stylistic impressions of particular artists, and care more about other abilities that remain (for now) the domain of the human artist, for example conceptual creativity or stylistic innovation.”
Turning to the benefits it could confer: what could DALL-E make easy that is currently very hard? In theory, systems like GPT-3 and DALL-E could be used in any domain where a problem is easy to formulate, but difficult to envision or impractical to implement.
NLPs can quickly process immense amounts of text, and a DALL-E variant could do the same for images. The use cases for this simple task are endless. Today, scholars, editors, and researchers in many fields lament that the rate of publishing is now too high for individuals to have a comprehensive understanding, or even a practicable overview, of their field.
The issue is: how can we safely incorporate artificial intelligence into consequential decision-making processes?
With the aid of powerful text and image comprehension tools, the scope of literary and historical research may suddenly expand exponentially (e.g. find all mentions of the phrase “silent as the grave” in English written history10). It can bootstrap research in science, whether the problem in question requires broad text search (e.g. conducting a thorough review of the literature for a specific topic) or the study and comparison of visual features (e.g. for taxonomists or anatomists, find all known images of dacetine ants).
Medicine and law are just two fields where the volume of reference material is immense, the time and resources of practitioners limited, and the decisions highly consequential. Could AI be of assistance here?
We can take an example from the field of radiology. Expert radiologists are able to detect “gists” of cancerous tissue years before the lesions characteristic of the disease become visible in mammogram screenings. Yet, such screenings yield false-positive and false-negative rates that are higher than what’s desirable. Currently, the development of AI tools to assist in the early detection of breast cancer is a field of active research and would ideally be used to complement human expertise.
Imagine now that a specially-trained version of DALL-E could be prompted to generate examples of what pre-cancerous breast tissue would look like for a given patient, provided with all the particularities relevant to the case. Such a tool could make explicit the cues that expert radiologists pick up on in their screenings, and provide a pedagogical tool for initial and ongoing training of practitioners. In the reverse direction, an image-processing system could scan for and raise issues about the screening. Such a failsafe could be integrated into standard office software, so as only to nudge human intuition if something has been detected by the engine but not yet addressed by the human user.
The promise of perfection in medical care is intoxicating. Yet, the thought experiment immediately raises a maze of ethical tripwires and new puzzles to solve: Can DALL-E’s images be considered a robust form of knowledge? Where and when can they be used to assist in making real world decisions? How do we implement safeguards into the underlying mechanisms that constrain the model in desirable ways without discouraging its “creativity”?
The issue, more broadly stated, is: how can we safely incorporate artificial intelligence into consequential decision-making processes?
Here, we return to the fundamental issue of alignment. We do not yet know how to effectively “teach” machines to reason in line with human interests and desires. This is also in part an impossible task, for we are frequently in the dark ourselves about where our motives lie and what trade-offs we are willing to make.
GPT-3, DALL-E, and related algorithms are promising tools, but which problems they are best suited to solving and how we can safely scale them for general use remains unclear.
Conclusions
In sum, DALL-E (and DALL-E 2) constitutes an impressive implementation of natural language processing and image generation models. Moreover, its surprising flexibility and “creativity,” given the poverty of the input it receives has generated much food for thought for AI researchers and the public alike. Yet, what tools like DALL-E can or should be used for in the wider world remains an open, important, and delicate question. For now, let us heed the example of the sorcerer’s apprentice, and play with caution.
Indeed, OpenAI’s DALL-E was quickly followed by the release of Google’s Imagen and, more recently, Parti. The technical strengths of these competing models aside, DALL-E has certainly won the meme war, being, at the timing of writing, far and away the most salient model shared on platforms like Twitter.
Refining the language of the text prompt given to the model through trial and error, to arrive at a version of the prompt the model “understands best,” or which provides the closest approximation of the intended result.
Reed et al. “Generative Adversarial Text to Image Synthesis,” Proceedings of the 33rd International Conference on Machine Learning, 2016
Perhaps a feature of its training set, or what it’s “learned,” DALL-E seems to be exceptionally good at portraying animals, particularly when asked to do so in an illustration.
Why asking for “a mouse riding a goldfinch” confused DALL-E while “an owl riding a Harley” didn’t is a mystery. Both prompts are fantastical, and owls on motorcycles seems hardly like something that it would be biased towards.
Many rendering problems seem to be solved by asking for an “oil canvas” version.
Note that DALL-E and DALL-E 2 use different underlying architectures. And while DALL-E uses systems similar to GPT-3, these are independent projects by OpenAI.
Incidentally, many have claimed that GPT-3 almost passes the Turing test, but not quite.
Arguably, this is evidence against its quality.
Google’s Ngram Viewer already permits the user to search for phrases in Google’s library of digitized books extending back to the year 1500. However, the program at present only works well for a few languages with larger corpora (English, French, German, Spanish, etc.) and is often stumped by calligraphic variations, particularly in older texts.